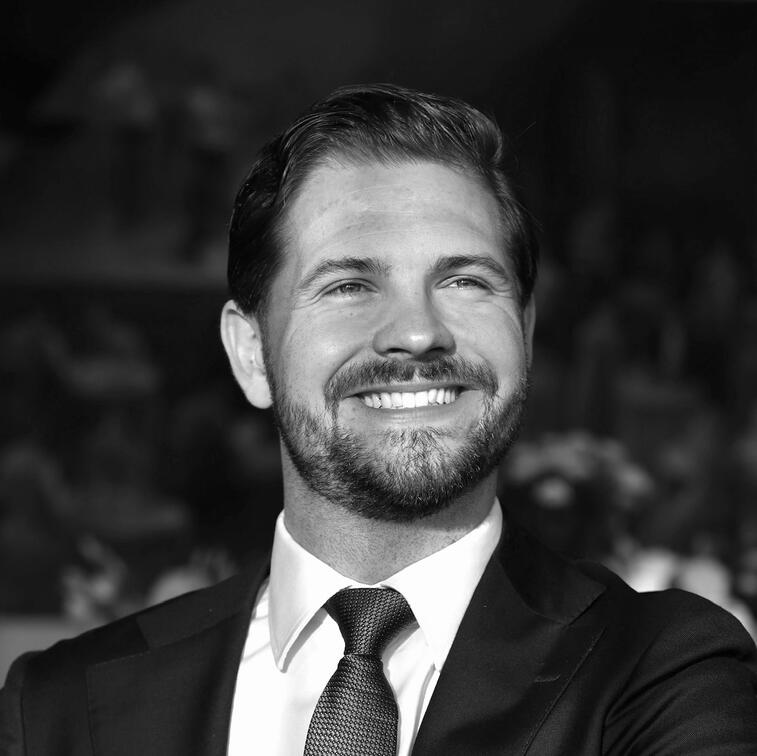
Hi!
I am Danish-American Ph.D. candidate in quantum physics. I am currently at the Center for Quantum Mathematics and Physics (QMAP) at UC Davis, studying under the supervision of Jaroslav Trnka. Before moving to California, I received my Bachelors and Masters degrees in Physics at the Niels Bohr Institute in Copenhagen. I love to solve hard problems using math and programming. My current research is in theoretical high energy physics, specifically in the geometric structure of scattering amplitudes. On this site you can find links to my research and other related works.
Navigate below to see a list of my publications and teaching experience.
Publications
Teaching
University of California, Davis
Winter 2024: Physics 155, lead TA -- General Relativity
Fall 2022: Physics 115A, lead TA -- Upper division Quantum Mechanics.
Spring 2022: Physics 105B, lead TA & 2 weeks of lecturing -- Upper division Classical Mechanics. I lectured on tidal effects, 4-vectors in special relativity, and general relativity.
8 Quarters spread over 2020-2024: Physics 9-series, discussion/lead TA. -- Introductory physics course for engineering students.
Summer 2021, Spring 2021, and Winter 2021: Physics 7-series, classroom/lab TA. -- The Physics 7-series is aimed towards introducing physics to non-physics majors, using inverted classroom methods.
Niels Bohr Institute
Spring 2020, Spring 2019, and Spring 2017: Thermodynamics -- discussion TA.
Fall 2019 and Fall 2018: Classical Mechanics -- discussion TA.
Summer 2018 and 2019: Kickstart -- discussion TA and organizer.
Spring 2019: Classical Mechanics -- re-exam workshop TA and organizer.
Spring 2018: Statistical Physics -- discussion TA.
Spring 2017: Electrodynamics -- discussion TA.
Fall 2016: Classical Mechanics -- lab TA.
Resume
CV
Click on images to download pdf
Quantum Physics and Mathematics (QMAP) graduate student journal club
I founded and run the student journal club. Below you can see previous and upcoming talks.
Fall 2024
Date: December 5, 2024
Speaker: Ben Eustis-Guthrie
Topic: Effective field theory corrections for latticesDate: November 26, 2024
Speaker: Robert Ferydouni
Topic: EntropyDate: November 21, 2024
Speaker: Melvyn Nabavi
Topic: The c-theoremDate: November 14, 2024
Speaker: Marcelo Dos Santos
Topic: Covariant quantization of the superparticleDate: November 7, 2024
Speaker: Hsing-Yi Lai
Topic: From classical to quantumDate: October 31, 2024
Speaker: Can Görmez
Topic: Quantization and geometryDate: October 24, 2024
Speaker: Umut Can Öktem
Topic: Strong coupling from perturbation theoryDate: October 17, 2024
Speaker: Artyom Lisitsyn
Topic: Constructing higher genus polylogarithms
Spring 2024
Date: May 20, 2024
Speaker: Robert Ferydouni
Topic: Lieb-Robinson bounds and applicationsDate: May 13, 2024
Speaker: Ben Eustis-Guthrie
Topic: Matrix product states and Hamiltonian truncationDate: May 6, 2024
Speaker: Robert Ferydouni
Topic: Solutions to Schrödinger Equation in Heisenberg pictureDate: April 29, 2024
Speaker: Siddharth Vadnerkar
Topic: Quantum computation using anyonsDate: April 22, 2024
Speaker: Ziyi Song
Topic: Conical geometries in AdS/CFTDate: April 15, 2024
Speaker: Subhobrata Chatterjee
Topic: Discrete dynamicsDate: March 18, 2024
Speaker: Hsing-Yi Lai
Topic: Local Lagrangian including magnetic chargesDate: March 11, 2024
Speaker: Christoph Bartch
Topic: Hidden zeros of scattering amplitudes